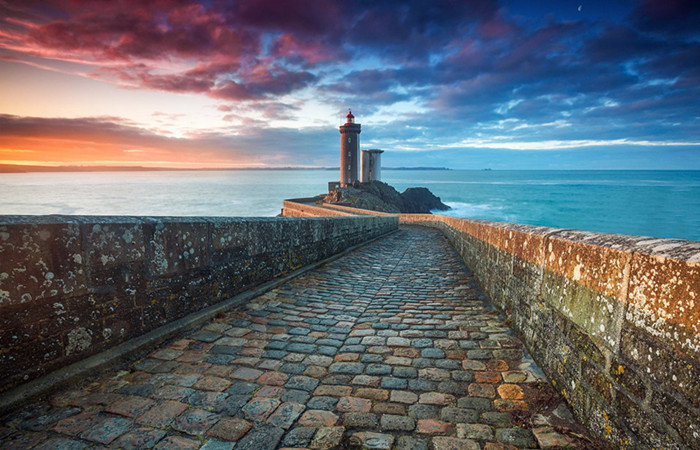
Python画图之Seaborn-1
声明:此文大部分样例及参考来源于科赛Kesci的公众号,学习后整理的学习笔记,特此声明。
seaborn官方网站:http://seaborn.pydata.org/examples/index.html ,提供了大量的gallery可直接替换到业务中使用
Seaborn是一个很棒的可视化库,尤其是当数据维度很大的时候,Seaborn可以让我们用最少的代码去绘制一些描述性统计的图,便于找寻各维度变量之间的特征,本篇我们将会利用科赛上Iris鸢尾花数据集。对Stripplot & Swarmplot, Boxplot & Violinplot, Barplot & Pointplot,以及抽象化的Factorplot,为大家进行讲解。
Iris鸢尾花数据集:Iris数据集是常用的分类实验数据集,由Fisher, 1936收集整理。“Iris也称鸢尾花卉数据集,是一类多重变量分析的数据集。数据集包含150个数据集,分为3类,每类50个数据,每个数据包含4个属性。可通过花萼长度(sepal_length),花萼宽度(sepal_width),花瓣长度(petal_length),花瓣宽度(petal_width)4个属性预测鸢尾花卉属于(Setosa,Versicolour,Virginica)三个种类中的哪一类”。
import warnings
warnings.filterwarnings("ignore")
import pandas as pd
import numpy as np
import matplotlib.pyplot as plt
import seaborn as sns
from sklearn.datasets import load_iris
#加载数据集
iris=load_iris()
iris1 = pd.DataFrame(iris.data)
iris1.columns = iris.feature_names
iris1.head()
sepal length (cm) | sepal width (cm) | petal length (cm) | petal width (cm) | |
---|---|---|---|---|
0 | 5.1 | 3.5 | 1.4 | 0.2 |
1 | 4.9 | 3.0 | 1.4 | 0.2 |
2 | 4.7 | 3.2 | 1.3 | 0.2 |
3 | 4.6 | 3.1 | 1.5 | 0.2 |
4 | 5.0 | 3.6 | 1.4 | 0.2 |
species=[]
for i in np.arange(len(iris.target)):
if iris.target[i]==0:
species.append(iris.target_names[0])
elif iris.target[i]==1:
species.append(iris.target_names[1])
else:
species.append(iris.target_names[2])
iris1['species'] = species
iris1.columns = ['sepal_length','sepal_width','petal_length','petal_width','species']
iris1.head()
sepal_length | sepal_width | petal_length | petal_width | species | |
---|---|---|---|---|---|
0 | 5.1 | 3.5 | 1.4 | 0.2 | setosa |
1 | 4.9 | 3.0 | 1.4 | 0.2 | setosa |
2 | 4.7 | 3.2 | 1.3 | 0.2 | setosa |
3 | 4.6 | 3.1 | 1.5 | 0.2 | setosa |
4 | 5.0 | 3.6 | 1.4 | 0.2 | setosa |
Stripplot:Stripplot的本质就是把数据集中具有Quantitative属性的变量按照类别去做散点图(Scatterplot)。
plt.figure(1,figsize=(12,6))
plt.subplot(1,2,1)
sns.stripplot(x='species',y='sepal_length',data=iris1) #stripplot
plt.title('Striplot of sepal length of Iris species')
with sns.axes_style("whitegrid"):
# 这个是临时设置样式的命令,如果不写,则按默认格式'darkgrid'进行绘制
plt.subplot(1,2,2)
plt.title('Striplot of sepal length of Iris species')
sns.stripplot(x='species',y='sepal_length',data=iris1,jitter=True) # jitterplot
plt.show()
上边左侧的图片便是在默认风格下用Stripplot绘制的散点图。在很多情况下,Stripplot中的点会重叠,使得我们不容易看出点的分布情况。一个简单的解决办法就是用在Stripplot的基础上绘制抖动图(jitterplot),仅沿着类别坐标轴的方向去随机微调整点的位置,显示出分布情况。
Swarmplot: 另一个解决Stripplot中点重叠的办法就是绘制Swarmplot,它的本质就是用通过算法,在类别坐标轴的方向上去‘延展’绘制这些原本重合的点。 我们将纸鸢花数据集中不同种类花的Petal Length和Petal width做Swarmplot可视化。
plt.figure(1,figsize=(12,6))
plt.subplot(1,2,1)
sns.swarmplot(x='species',y='petal_length',data=iris1)
with sns.axes_style("ticks"): # 这次使用了ticks风格
plt.subplot(1,2,2)
sns.swarmplot(x='species',y='petal_width',data=iris1)
plt.show()
Boxplot: 箱形图,主要包含六个数据节点,将一组数据从大到小排列,分别计算出上边缘,上四分位数Q3,中位数,下四分位数Q1,下边缘,还有异常值。 下面将纸鸢花数据集中的四个变量sepal_length, sepal_width, petal_length和petal_width做箱形图可视化。
var = ['sepal_length','sepal_width','petal_length','petal_width']
axes_style = ['ticks','white','whitegrid', 'dark']
fig = plt.figure(1,figsize=(12,12))
for i in range(4):
with sns.axes_style(axes_style[i]):
# 将除了默认的darkgrid之外的样式都展现一遍
plt.subplot(2,2,i+1)
sns.boxplot(x='species',y=var[i],data=iris1)
plt.show()
Violinplot: Violinplot相当于结合了箱形图与核密度图,更好地展现出数据的量化形态。
context= ['notebook','paper','talk','poster']
axes_style = ['ticks','white','whitegrid', 'dark']
plt.figure(1,figsize=(12,12))
for i in range(4):
with sns.axes_style(axes_style[i]):#设置axes_style
sns.set_context(context[i])
# 设置context style,默认为notebook,除此之外还有paper,talk,poster
plt.subplot(2,2,i+1)
plt.title(str(var[i])+ ' in Iris species')
sns.violinplot(x='species',y=var[i],data=iris1)
plt.show()
Violinplot用Kernel Density Estimate去更好地描述了quantitative变量的分布。
与此同时,也可以组合Swarmplot和Boxplot或Violinplot去描述Quantitative变量。用鸢尾花数据集展示如下:
context= ['notebook','paper','talk','poster']
axes_style = ['ticks','white','whitegrid', 'dark']
plt.figure(1,figsize=(12,12))
for i in range(4):
with sns.axes_style(axes_style[i]):#设置axes_style
sns.set_context(context[i])#设置context
plt.subplot(2,2,i+1)
plt.title(str(var[i])+ ' in Iris species')
sns.swarmplot(x='species', y=var[i], data=iris1, color="w", alpha=.5)
if i % 2 == 0:
sns.violinplot(x='species', y=var[i], data=iris1, inner=None)
else:
sns.boxplot(x='species', y=var[i], data=iris1)
# 分别用swarmplot+violinplot 和swarmplot + boxplot
plt.show()
Barplot: Barplot主要是展现在分类中的Quantitative变量的平均值情况,并且用了Boostrapping算法计算了估计值的置信区间和Error bar.用鸢尾花数据集
plt.figure(1,figsize=(12,12))
for i in range(4):
with sns.axes_style(axes_style[i]):#设置axes_style
sns.set_context(context[i])
# 设置context style,默认为notebook,除此之外还有paper,talk,poster
plt.subplot(2,2,i+1)
plt.title(str(var[i])+ ' in Iris species')
sns.barplot(x='species',y=var[i],data=iris1)
plt.show()
Countplot: 如果想知道在每个类别下面有多少个观察值,用Countplot就可以,相当于是做一个Observation Counts,用鸢尾花数据集展示如下:
plt.figure(figsize=(5,5))
sns.countplot(y="species", data=iris1) # 设置y='species',将countplot水平放置
plt.title('Iris species count')
plt.show()
Pointplot: Pointplot相当于是对Barplot做了一个横向延伸,一方面,用Point Estimate和Confidence Level去展示Barplot的内容;另一方面,当每一个主类别下面有更细分的Sub-Category的时候,Pointplot可以便于观察不同Sub-Category在各主类别之间的联系。展示如下:
plt.figure(1,figsize=(14,14))
for i in range(4):
with sns.axes_style(axes_style[i]):#设置axes_style
sns.set_context(context[i])
# 设置context style,默认为notebook,除此之外还有paper,talk,poster
plt.subplot(2,2,i+1)
plt.title(str(var[i])+ ' in Iris species')
sns.pointplot(x='species',y=var[i],data=iris1)
plt.show()
Factorplot: Factorplot可以说是Seaborn做Category Visualization的精髓,前面讲的这些Plot都可以说是Factorplot的具体展示。我们可以用PariGrid去实现对多个类别的数值特征用同一种Plot做可视化。
sns.set(style="ticks")
g = sns.PairGrid(iris1,x_vars = ['sepal_length','sepal_width','petal_length',
'petal_width'],
y_vars = 'species', aspect=0.75,size=4)
# 设置间距和图片大小
g.map(sns.violinplot,palette='pastel')
plt.show()